If you haven't heard of p-hacking, it's as follows: suppose you want to find predictors for cancer. You test 100 possible predictors each at p-value 5%. Now although the chance of a false positive on any given test is 5%, you're expected to get 5 false positives in the test. So you can "always" find a (fake) predictor for cancer just by surveying enough things.
Another way of putting it: even if your factors don't actually predict cancer, the probability distribution for the observed correlation for any one factor may look something like this.
So when you test for these factors, when the correlations you observe actually sample the above curve, you're faced with a question: do you believe the points that lie in the shaded region (beyond your significance level) actually reject the null hypothesis?
On one hand: they lie beyond your significance level. Individually, you need to reject those null hypotheses.
On the other hand, one can also think of a "mega-" null hypothesis as implying the above curve: since your points sample the curve, you need to accept the null hypothesis.
I believe the answer is to reject those null hypotheses, i.e. to not make any "corrections" for having tested multiple parameters.
Here are some explanations:
- An individual researcher "p-hacking" is fundamentally/mathematically no different from a large number of researchers investigating of various different parameters. Surely it makes no sense to argue that all positive results in the literature should be ignored, or that they should be evaluated at much stronger significance levels.
- When you investigate a large number of parameters, the probability of a true positive is also higher (in a Bayesian sense). If your positives are more likely to be false than true when you're testing a hundred parameters, they were more likely to be false than true when testing one parameter too. Of course, you are much more likely to have false positives when testing more parameters, but that doesn't increase the chance that any given deduction is false, because there are more true positives, too.
- Or in other words, the "mega-null hypothesis" probably isn't true. If the $\theta$ parameters are independent, then you'll probably have a large number of false null hypotheses. The "mega-null hypothesis" argument actually seems to assume zero probability of a true positive.
- Equivalently: just apply Bayes's theorem/the fact that probability is commutative. (equivalent to the first point)
Also note how the Bonferroni correction has nothing to do with p-hacking: it applies to looking at probabilities/confidence levels of several hypotheses being true, not about one.
So, then, why do the consequences of p-hacking all seem to bizarre? Stuff like this:
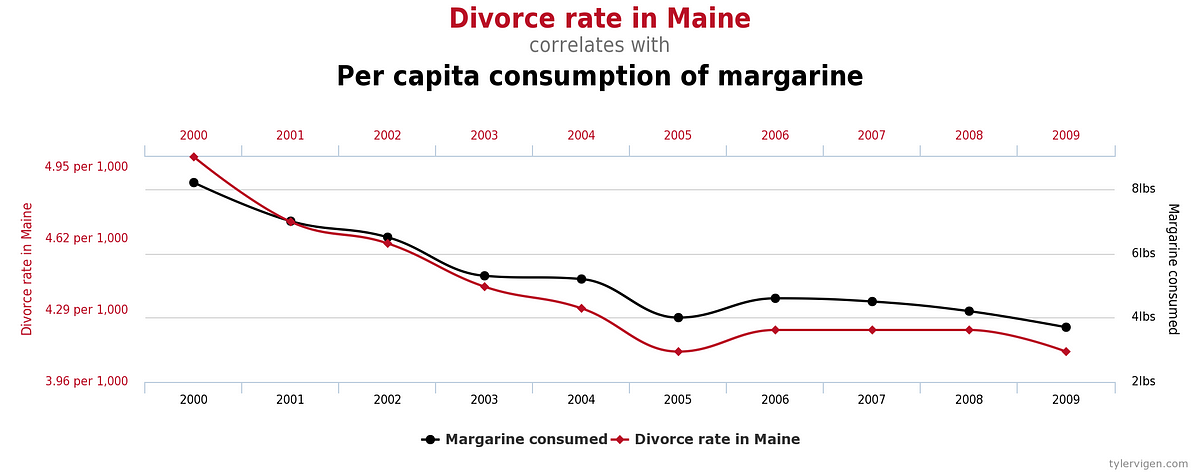
Or this:
![]() |
Full comic for context: xkcd 882 |
And no, we don't.
Why not?
Because there is no a priori reason to suspect that margarine causes divorces in Maine. Because a priori, we know that it's highly likely that whether green jelly beans cause acne is correlated with whether all the other color of jelly beans cause acne (because it's very unlikely that colour itself causes acne). These correlations should be embedded in our prior.
But these aren't fundamental issues with the nature of statistics. These are issues with how frequentist researchers may often decide which hypotheses to test. One should have some theoretical justification to formulate a hypothesis: that's how you know the prior probability is significant. Unless you have a good theoretical model for why a certain correlation/etc. should hold, the hypothesis should not be tested.
This problem is particularly prevalent in the social sciences, where a "general mathematical theory" of social science does not exist. Even in economics, you often end up with pseudo-science like this: Want to expand the economy? Tax the rich! (this particular study was terrible on several levels: (1) the conflation of correlation and causation -- this is always a problem when you have temporal trends, because time is a hidden parameter; that's why you should do cross-sectional studies (2) the correlation was statistically insignificant by any standard (3) there was no theoretical justification for why progressive taxation would expand the economy, leading to the problems discussed in this post.)
This point is essentially the point made by several papers (links: [1][2]) discussing "multiple comparisons in a Bayesian setting" -- it is what is meant by claims like "the multiple comparisons problem disappears when you use a hierarchial Bayesian model with correlations between your parameters".
No comments:
Post a Comment